Law of Large Numbers — More Numbers More Statistical Stability
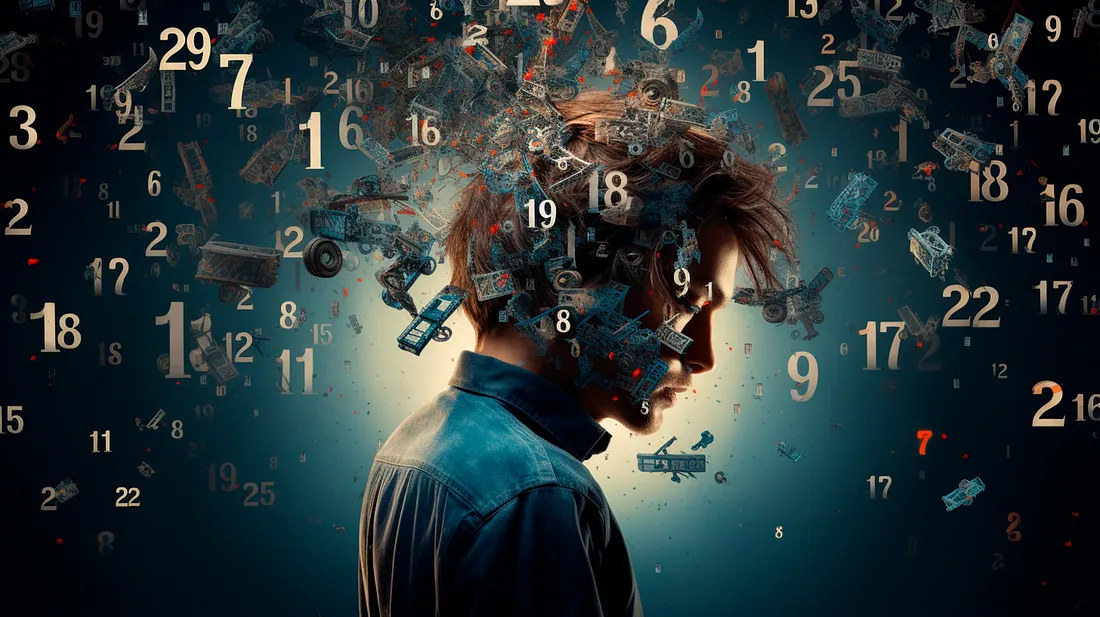
The Essence of the Law of Large Numbers
The Law of Large Numbers is a fundamental principle in statistics that describes the behavior of sample averages as the sample size increases. Simply put, as we collect more data, our estimates tend to get closer to the true population parameter.
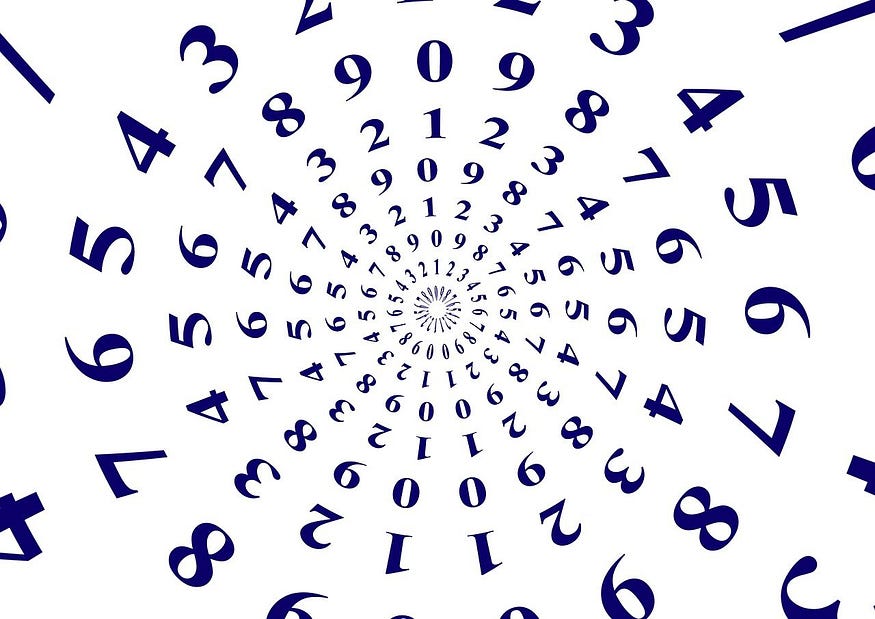
This was then formalized as a law of large numbers. A special form of the LLN (for a binary random variable) was first proved by Jacob Bernoulli. It took him over 20 years to develop a sufficiently rigorous mathematical proof which was published in his Ars Conjectandi (The Art of Conjecturing) in 1713. He named this his “Golden Theorem” but it became generally known as “Bernoulli’s theorem”.
In 1837, S. D. Poisson a French statistician further described it under the name “la loi des grands nombres” (“the law of large numbers”).
After Bernoulli and Poisson published their efforts, other mathematicians also contributed to the refinement of the law, including Chebyshev, Markov, Borel, Cantelli, Kolmogorov, and Khinchin.
Markov further’s studies have given rise to two prominent forms of the LLN. One is called the “weak” law and the other the “strong” law.
Let’s quickly start with an example:
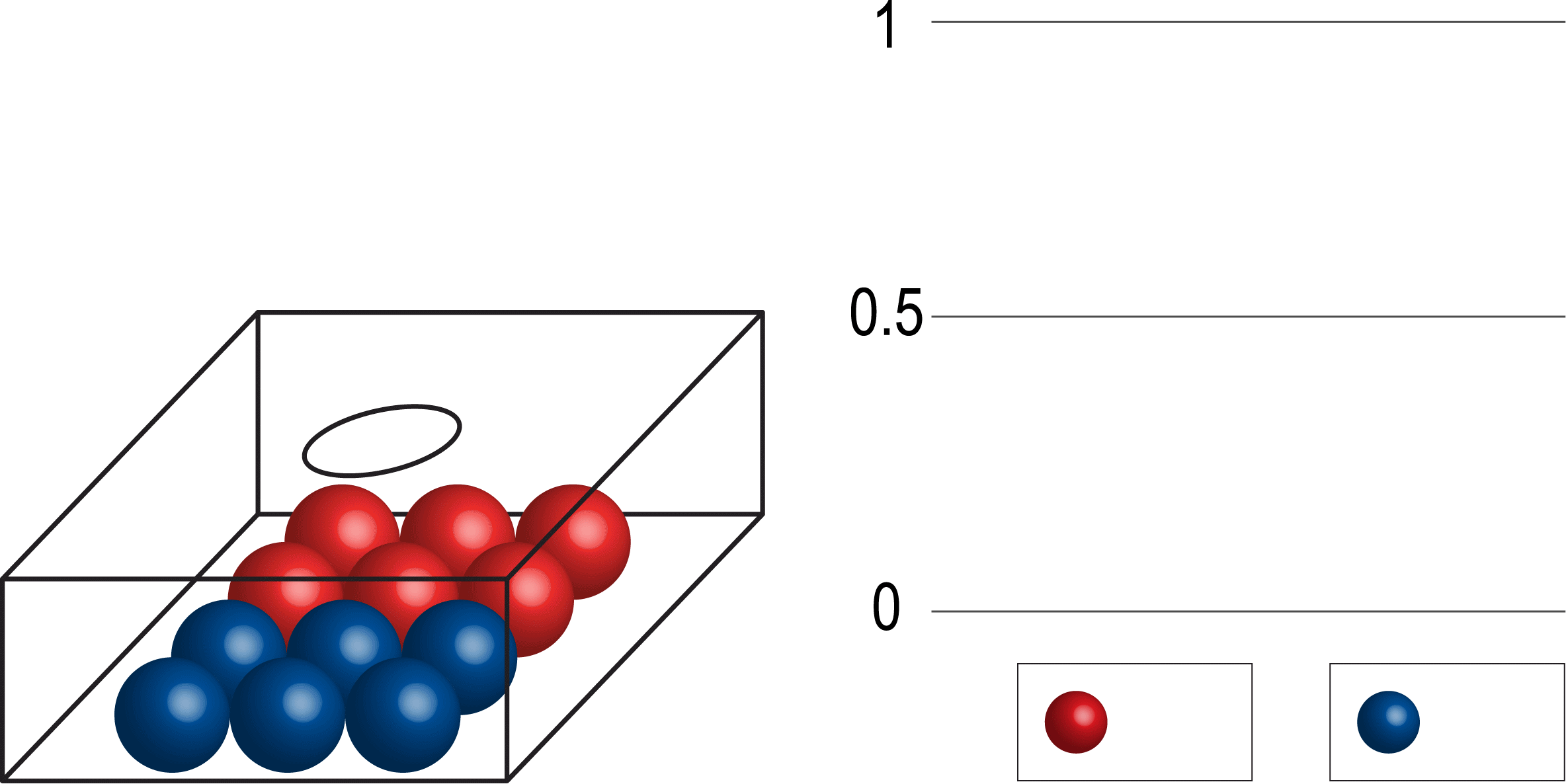
Even if you started by picking a bunch of blue marbles in a row, eventually, as you keep picking, the ratio of blue to red will start to even out.
So, in essence, the law of large numbers tells us that with a large enough sample size, the observed outcomes will more closely reflect the true underlying probabilities.
Hakuna Matata Meaning :-“As we increase the sample size the sample mean approaches the population mean’’The more data we have, the more reliable our estimates become.
Why It Matters?
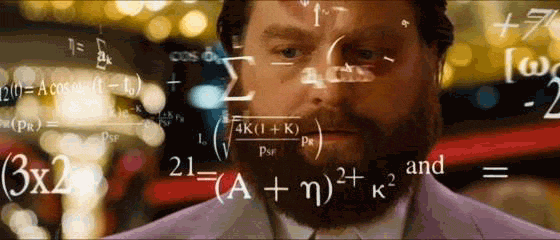
It’s a bit like saying that the more you practice something, the better you’ll get at it. So, whether you’re flipping coins, rolling dice, or analyzing data, knowing about the Law of Large Numbers can help you understand how reliable your results are.
There are plenty of examples of this Law:
Example — 1:- Stocks
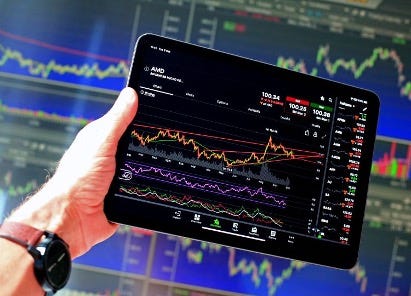
Example — 2:- Healthcare
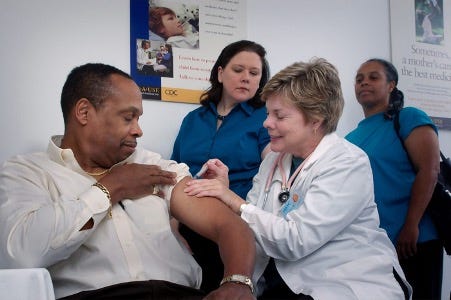
Don’t worry it takes time so here is my favorite Example:
Let’s consider the example of Exit polls in an election: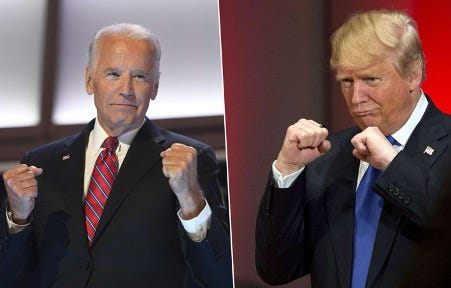
This means that with a sufficiently large sample size, the exit polls are more likely to accurately reflect the actual voting patterns of the entire electorate.
So, the law of large numbers assures us that as the sample size increases, the exit poll results will converge towards the expected outcome, providing a more reliable indication of the election results.
Do you know why Casino is mostly in profits? 😂
A casino may lose money in a single spin of the roulette wheel, its earnings will tend towards a predictable percentage over a large number of spins.Precaution:-
- There is always a chance that the actual result will be very different from the expected value, even with a large number of trials. For example, if a coin is flipped 1,000 times, it is still possible to get 600 heads and 400 tails, even though the expected value is 500 heads and 500 tails.
- The Law of Large Numbers is often mistaken for the belief that past outcomes will affect future events. This is not the case this is the assumption that each event is independent and unaffected by the outcomes of previous events. For example, if a coin has landed on heads for the past 10 flips, the probability of it landing on heads again is still 50/50.
ABOUT THE AUTHOR
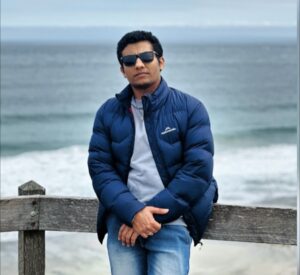
Harshit Sanwal
Marketing Analyst, DataMantra